A few days ago, the Alibaba research team built a method called Animate Anyone, which only requires a photo of a person and is guided by skeletal animation to generate a natural animated video. However, the source code for this study has not yet been released.
Let Iron Man move.
In fact, the day before the paper Animate Anyone appeared on arXiv, the National University of Singapore Show Laboratory and Byte jointly conducted a similar study. They proposed MagicAnimate, a diffusion-based framework designed to enhance temporal consistency, faithfully preserve reference images, and improve animation fidelity. Moreover, the MagicAnimate project is open source, and the inference code and gradient online demo have been released.
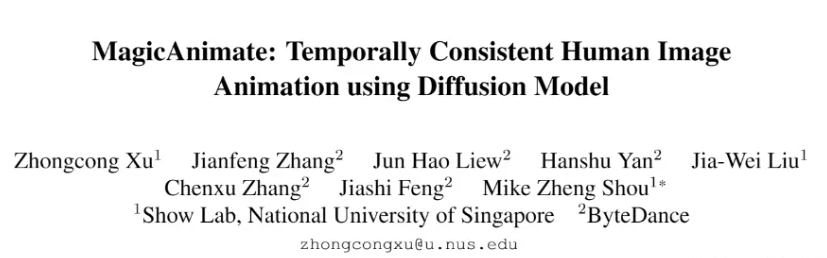
- Paper address: arxiv.org/pdf/2311.16…
- Project address: showlab.github.io/magicanimat…
- GitHub address: github.com/magic-resea…
To achieve the above goals, the researchers first developed a video diffusion model to encode temporal information. Then to maintain appearance coherence across frames, they introduce a novel appearance encoder to preserve the complex details of the reference image. Taking advantage of these two innovations, the researchers further used simple video fusion technology to ensure smooth transitions of long video animations.
Experimental results show that MagicAnimate outperforms the baseline method on both benchmarks. Especially on the challenging TikTok dancing data set, our method outperforms the strongest baseline method by more than 38% in video fidelity.
Let’s take a look at the dynamic display effects of the following TikTok ladies.
In addition to the dancing TikTok ladies, there is also Wonder Woman who “runs”.
The Girl with a Pearl Earring and the Mona Lisa both did yoga.
In addition to being a single person, dancing with multiple people can also be done.
Compared with other methods, the effect is superior.
Some foreign netizens have created a trial space on HuggingFace, and it only takes a few minutes to create an animated video. But this website has already received a 404.
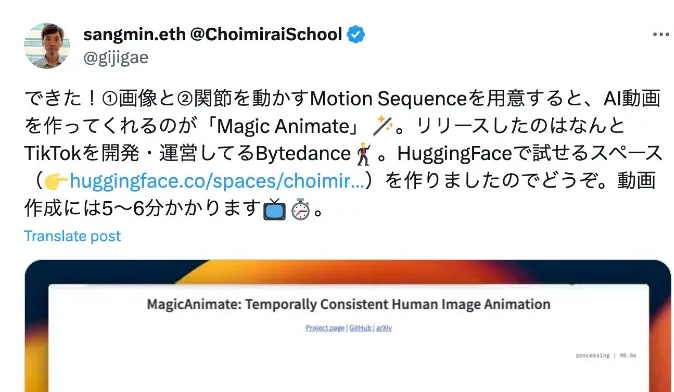
Source: twitter.com/gijigae/sta…
Next, we introduce the MagicAnimate method and experimental results.
Method overview
Given a reference image I_ref and a motion sequence , where N is the number of frames. MagicAnimate is designed to synthesize continuous video
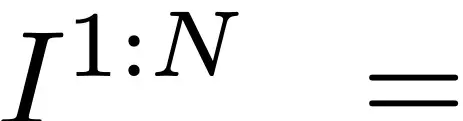
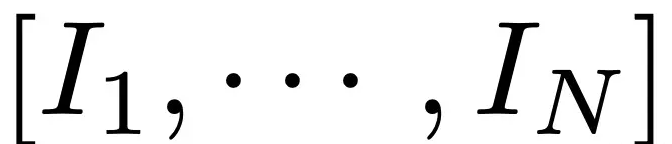
. The picture I_ref appears in it, while following the motion sequence
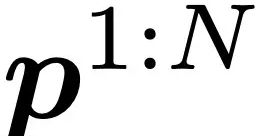
. Existing frameworks based on the diffusion model process each frame independently, ignoring the temporal consistency between frames, resulting in a “flickering” problem in the generated animation.
To solve this problem, this study builds a video diffusion model for temporal modeling by incorporating a temporal attention block into a diffusion backbone network .
In addition, existing works use CLIP encoders to encode reference images, but this study believes that this method cannot capture complex details. Therefore, this study proposes a new appearance encoder to encode I_ref into the appearance embedding y_a, and adjust the model based on this.
The overall process of MagicAnimate is shown in Figure 2 below. First, the appearance encoder is used to embed the reference image into the appearance embedding, and then the target pose sequence is passed to the pose ControlNet to extract motion conditions
.
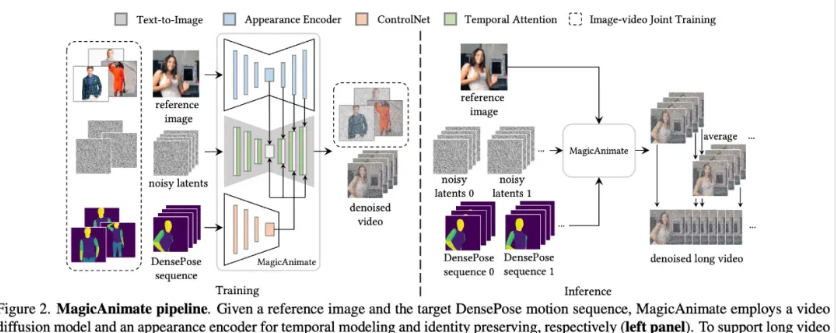
In practice, due to memory constraints, MagicAnimate processes the entire video in segments. Thanks to temporal modeling and powerful appearance encoding, MagicAnimate maintains a high degree of temporal and appearance consistency between clips. But there are still subtle discontinuities between parts, and to alleviate this, the research team used a simple video fusion method to improve transition smoothness.
As shown in Figure 2, MagicAnimate decomposes the entire video into overlapping segments and simply averages the predictions of overlapping frames. Finally, this study also introduces an image-video joint training strategy to further enhance the reference image retention capability and single-frame fidelity.
Experiments and results
In the experimental part, the researchers evaluated the performance of MagicAnimate on two data sets, namely TikTok and TED-talks. The TikTok data set contains 350 dancing videos, and TED-talks contains 1,203 clips extracted from TED talk videos on YouTube.
Let’s look at the quantitative results first. Table 1 below shows the quantitative results comparison between MagicAnimate and baseline methods on the two data sets. Table 1a shows on the TikTok data set that our method surpasses all baseline methods in reconstruction indicators such as L1, PSNR, SSIM and LPIPS.
Table 1b shows that on the TED-talks dataset, MagicAnimate is also better in terms of video fidelity, achieving the best FID-VID score (19.00) and FVD score (131.51).
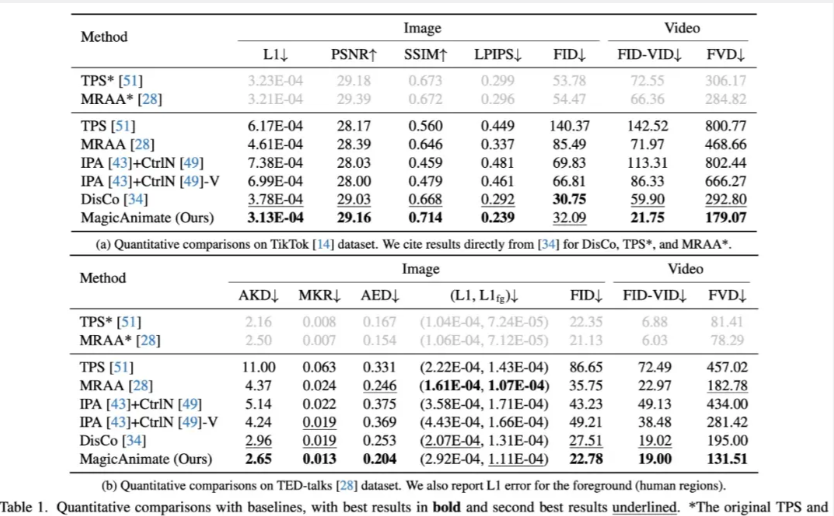
Let’s look at the qualitative results again. The researchers show a qualitative comparison of MagicAnimate with other baseline methods in Figure 3 below. Our method achieves better fidelity and exhibits stronger background preservation, thanks to the appearance encoder that extracts detail information from the reference image.
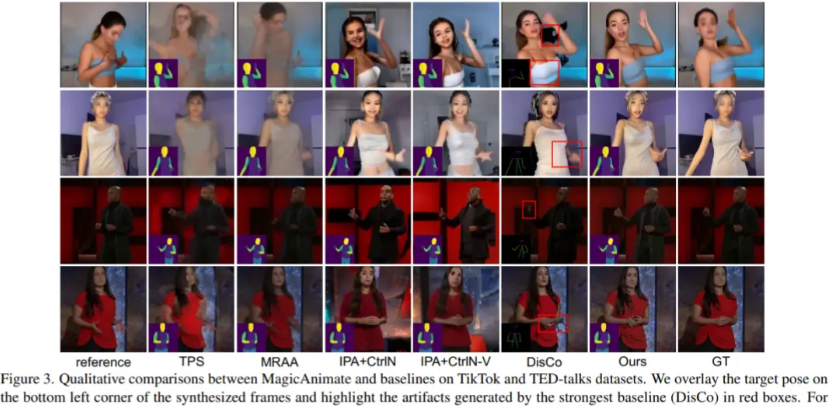
The researchers also evaluated MagicAnimate’s cross-identity animation and compared it with SOTA baseline methods, namely DisCo and MRAA. Specifically, they sampled two DensePose motion sequences from the TikTok test set and used these sequences to animate reference images from other videos.
Figure 1 below shows that MRAA cannot generalize to driving videos containing a large number of different poses, while DisCo has difficulty retaining details in the reference image. In contrast, our method demonstrates its robustness by faithfully animating a reference image for a given target motion.
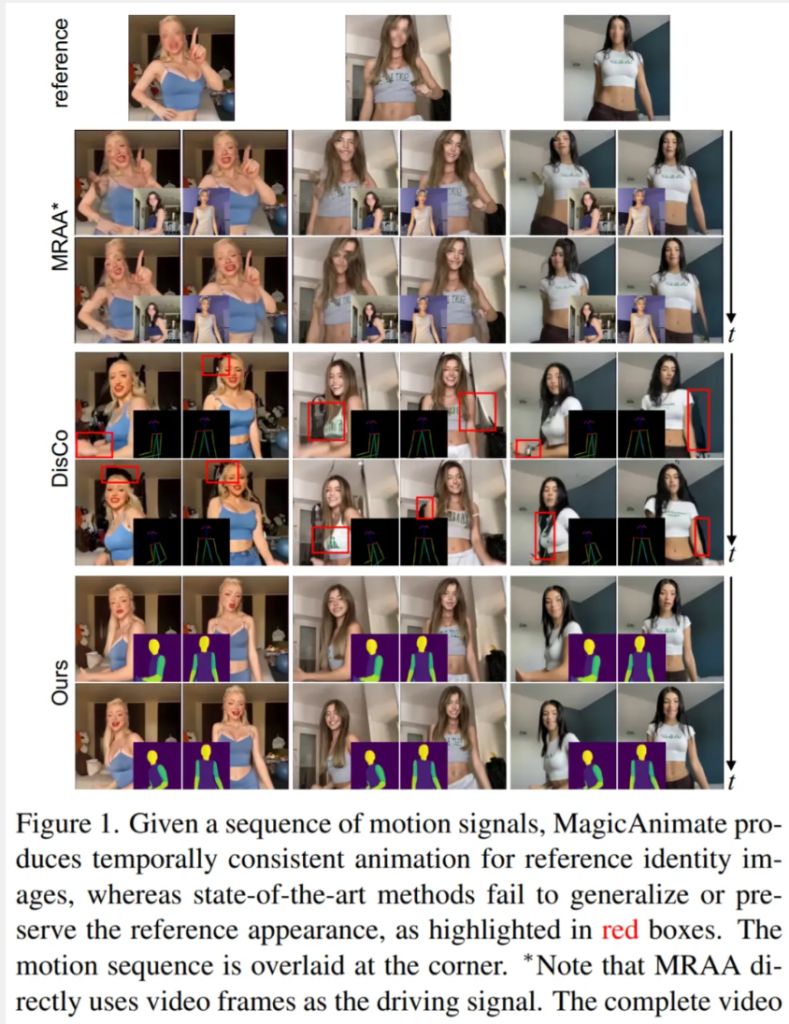
Finally, there is the ablation experiment. In order to verify the effectiveness of the design choices in MagicAnimate, the researchers conducted ablation experiments on the TikTok data set, including with and without temporal modeling, appearance encoder, inference stage video fusion, and image-video joint as shown in Table 2 below and Figure 4 below. training etc.
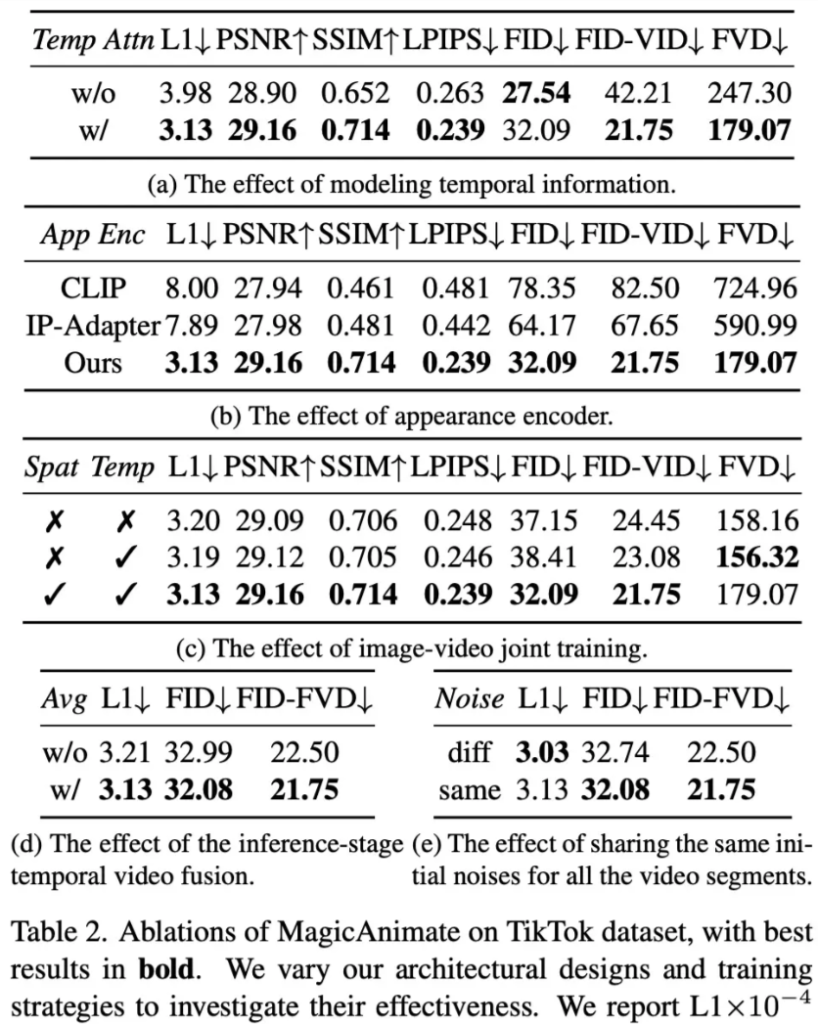
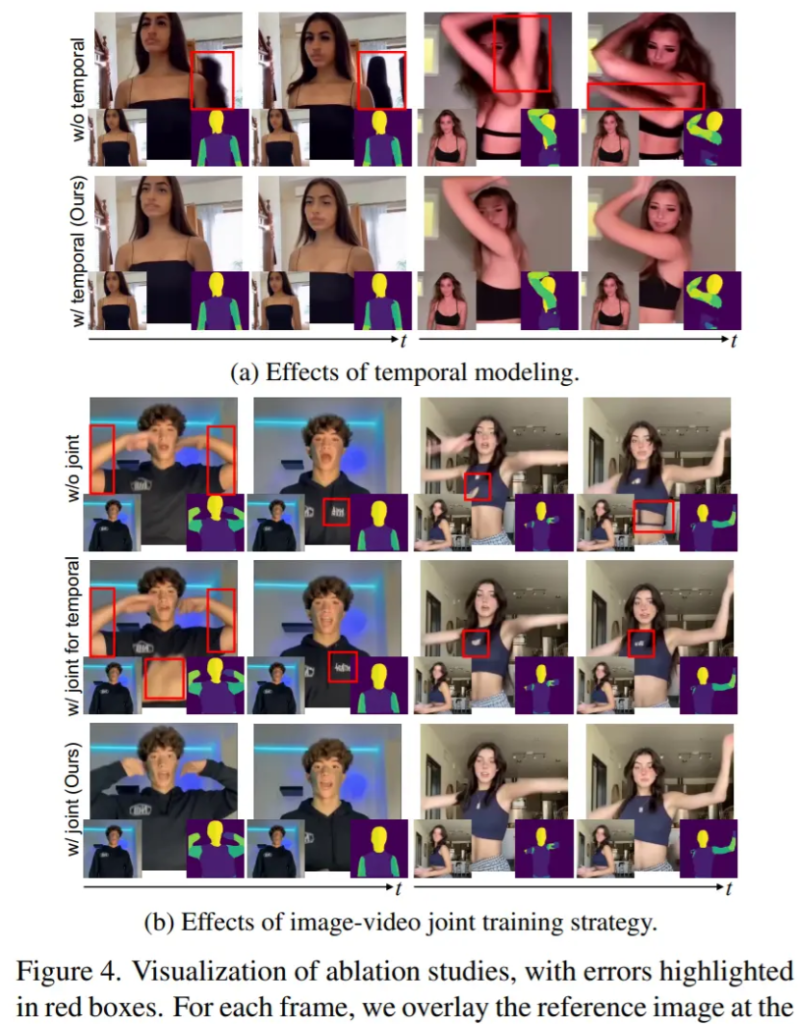
MagicAnimate also has broad application prospects. The researchers say that despite being trained only on real human data, it has demonstrated the ability to generalize to a variety of application scenarios, including animation of unseen domain data, integration with text-image diffusion models, and Multiplayer animation, etc.