In the field of artificial intelligence, the generation modeling of video data has always been a very challenging and innovative research direction. From recurrent networks to generative adversarial networks, to autoregressive transformers and diffusion models, countless attempts have shown us the rapid advancement of this technology. Now, OpenAI has brought its latest research results- Sora video generation model, which redefines our understanding of AI-generated content .
You can’t just wait and see AIGC, and follow the trend to party, you should go in to understand it and become its master!
Today, let’s take a deeper look at the technology behind Sora based on the official OpenAI article.
Sora is more than a model, it is a world simulator
According to the official website of OpenAI , Sora is not only a universal visual data model, it is also a world simulator . With the ability to generate videos and images of varying durations, aspect ratios and resolutions, Sora can produce high-definition videos up to one minute, ushering in a new era in video generation.
Sora’s core strength lies in its high degree of adaptability and diversity. Whether it’s a short animation or a complex and rich scene video, Sora can create it through simple text prompts. The introduction of this technology provides great convenience to content creators, while also expanding the possibilities of simulating the real world.
This is the Sora video officially released by OpenAI, which is 55s long: openai.com/research/vi…
The technology behind Sora
There were many video generation models before Sora, such as Runway and PiKa . These works usually focused on a narrow range of visual data, shorter videos or fixed-size videos . Sora is a universal visual data model – it can generate videos and images of varying durations, aspect ratios and resolutions, up to a minute of high-definition video .
What’s the secret behind it?
Turning visual data into patches
Large-scale language models gain powerful capabilities by training extremely large-scale data, and the extent of these language models is largely due to Token.
Based on this inspiration, Sora thus uses visual patches . At a high level, we first convert the video into small patches by compressing the video into a low-dimensional latent space, and then decompose this representation into spatiotemporal patches.
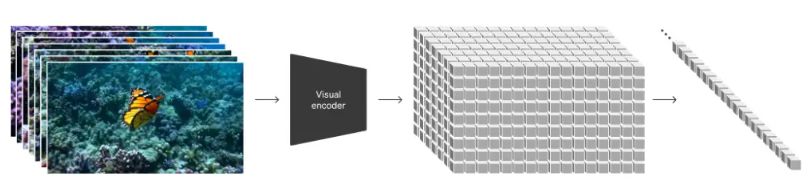
Video Compression Network
The network converts the original video into a compressed latent representation through dimensionality reduction techniques. This network enables the model to operate in a simpler space, improving efficiency when processing videos.
Spacetime Latent Patches
The Sora model divides the video into a series of spatiotemporal patches, which serve as tokens for the transformer network. The patching processing method provides an effective visual data representation and facilitates the network to capture the spatiotemporal characteristics of the video.
Video Scaling Transformers
Transformers networks have demonstrated outstanding performance in many areas such as language, vision, and audio processing. Sora uses this architecture to extend video generation capabilities. These transformers are trained to predict original “clean” patches, resulting in clean video output.
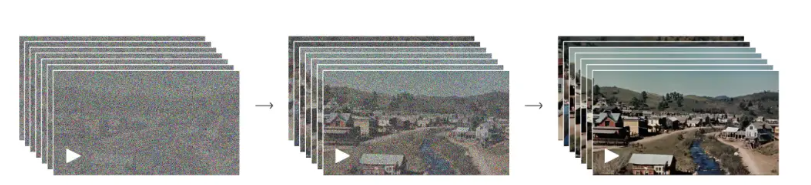
Conditional Diffusion Models
As a diffusion model, Sora generates videos based on input noise patches and conditional information (such as text cues). In this way, Sora is able to gradually generate clear and meaningful video content from inputs with a certain degree of randomness.
And as the amount of training calculations increases, the sample quality improves significantly, as shown in the figure below.
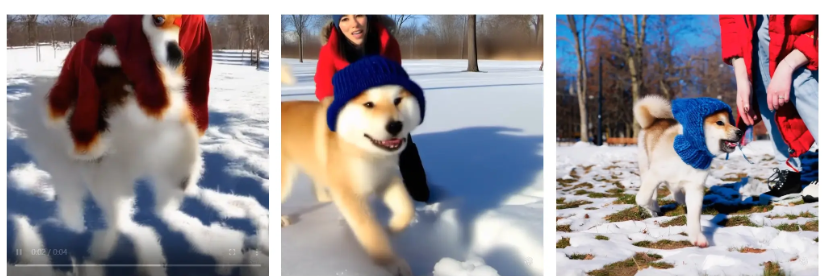
Variable Durations, Resolutions, Aspect Ratios
Past methods of generating images and videos typically resize, crop, or crop the video to a standard size—for example, a 4-second long video with a resolution of 256×256. We found that training on the data at its original size brings several benefits.
Sora does not limit the size of videos when training, so it can generate videos of various sizes. This means that Sora can not only generate standard-sized videos, but also create more diverse video formats to suit different playback devices and uses.
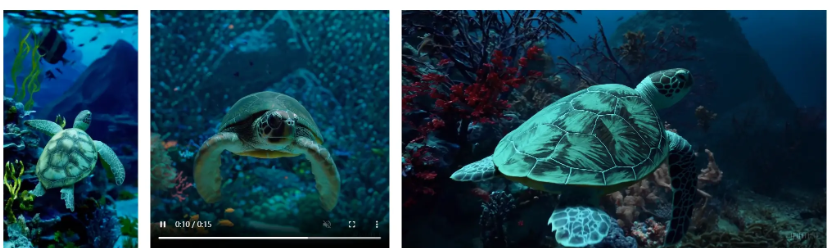
Overall, this allows Sora to achieve flexible sampling and improve composition, ultimately achieving good video effects.
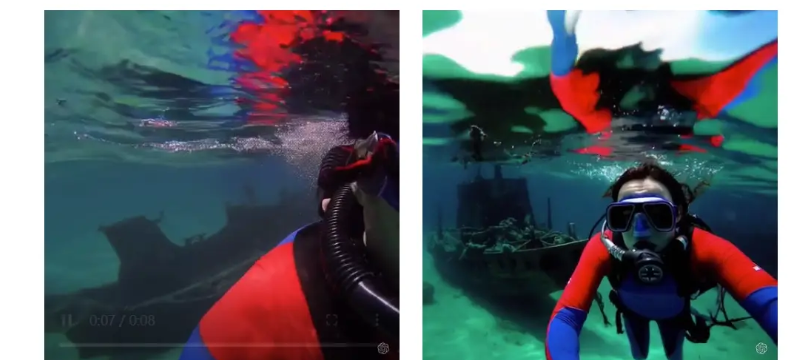
Language Understanding
Training text-to-video generation systems requires a large number of videos with corresponding text captions. OpenAI applies the re-annotation technology introduced in DALL·E 3 to videos. First train a highly descriptive annotation model and then use it to generate textual captions for all videos in the training set. The results of the study found that training on highly descriptive video titles improved text accuracy as well as the overall quality of the video. Sora is trained using highly descriptive video titles to improve text understanding. Similar to DALL·E, Sora can also convert short user prompts into more detailed instructions, improving the consistency of video output and text prompts .
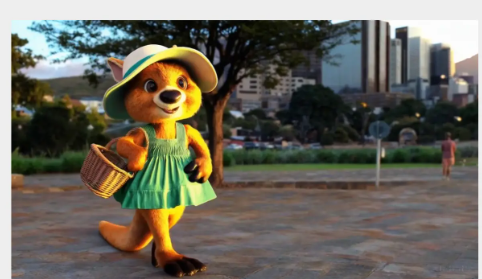
Through the combination of these advanced technologies, the Sora model is able to generate high-quality video content while making significant progress in the efficiency and diversity of video generation.
Looking to the future
As a simulator, Sora has limitations in demonstrating its capabilities, but it has shown that expanding the scale of video models is a promising development direction. Its emerging capabilities demonstrate the potential to simulate both the real and digital worlds, while simulating physical interactions still needs refinement.
In the field of AI video, the launch of the Sora model is indeed a milestone event . With the iterative updates of technology, we have reason to expect that Sora will bring us a more realistic and immersive digital experience in the near future. Let’s wait and see, more may be unlocked.
Conclusion
Stay curious and keep exploring!
AI is an opportunity for all of us, grab it!