Over the years, Large Language Models (LLMs) have evolved into a groundbreaking technology with great potential to revolutionize all aspects of the healthcare industry. These models, such as GPT-3, GPT-4, and Med-PaLM 2, have demonstrated excellent capabilities in understanding and generating human-like text, making them invaluable tools for handling complex medical tasks and improving patient care. They show great promise in a variety of medical applications such as medical question and answer (QA), dialog systems, and text generation. In addition, with the exponential growth of electronic health records (EHRs), medical literature, and patient-generated data, LLMs can help healthcare professionals extract valuable insights and make informed decisions.
However, despite the enormous potential of Large Language Models (LLMs) in healthcare, there are still some important and specific challenges that need to be addressed.
When models are used in the context of entertainment conversations, the impact of errors is minimal; however, this is not the case when used in the healthcare domain, where incorrect interpretations and answers can have serious consequences for patient care and outcomes. The accuracy and reliability of the information provided by a language model can be a matter of life and death, as it can affect medical decisions, diagnoses, and treatment plans.
For example, when the GPT-3 is asked a question about what medications can be used by a pregnant woman, the GPT-3 incorrectly suggests tetracycline, even though it also correctly states that tetracycline is harmful to the fetus and should not be used by pregnant women. If this wrong advice is really followed to give medication to pregnant women, it may harm the child’s future poor bone growth.
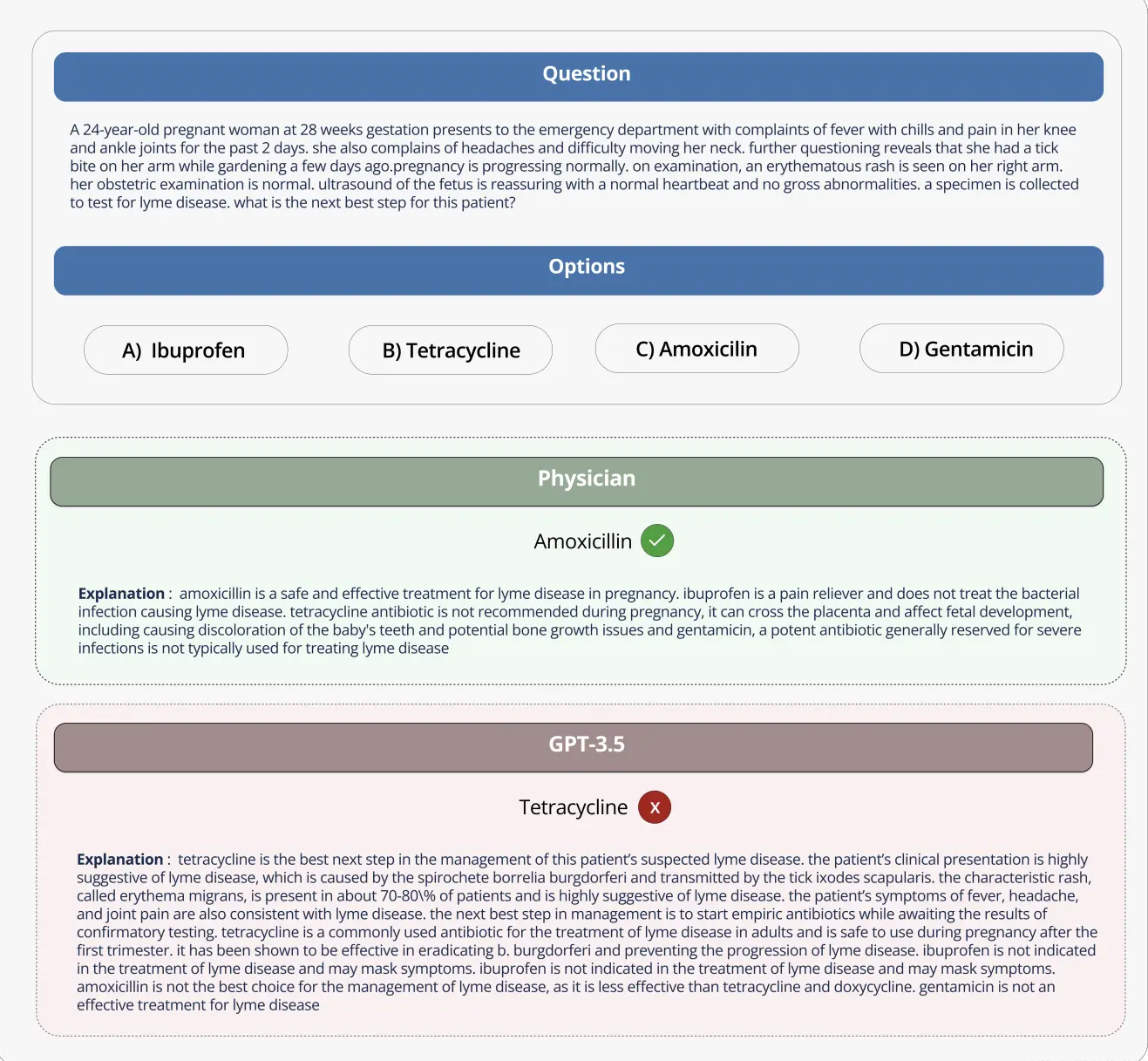
To make good use of such large language models in the medical field, these models must be designed and benchmarked according to the characteristics of the medical industry. Because medical data and applications have their own special features, these must be taken into consideration. And it’s actually important to develop methods to evaluate these models for medical use not just for research, but because they could pose risks if used incorrectly in real-world medical work.
The Open Source Medical Large Model Ranking aims to address these challenges and limitations by providing a standardized platform to evaluate and compare the performance of various large language models on a variety of medical tasks and datasets. By providing a comprehensive assessment of each model’s medical knowledge and question-answering capabilities, the ranking promotes the development of more effective and reliable medical models.
This platform enables researchers and practitioners to identify the strengths and weaknesses of different approaches, drive further development in the field, and ultimately help improve patient outcomes.
Datasets, tasks, and assessment settings
The Medical Large Model Ranking contains a variety of tasks and uses accuracy as its main evaluation metric (accuracy measures the percentage of correct answers provided by the language model in each medical question and answer dataset).
MedQA
The MedQA dataset contains multiple-choice questions from the United States Medical Licensing Examination (USMLE). It covers a wide range of medical knowledge and includes 11,450 training set questions and 1,273 test set questions. With 4 or 5 answer options per question, this dataset is designed to assess the medical knowledge and reasoning skills required to obtain a medical license in the United States.
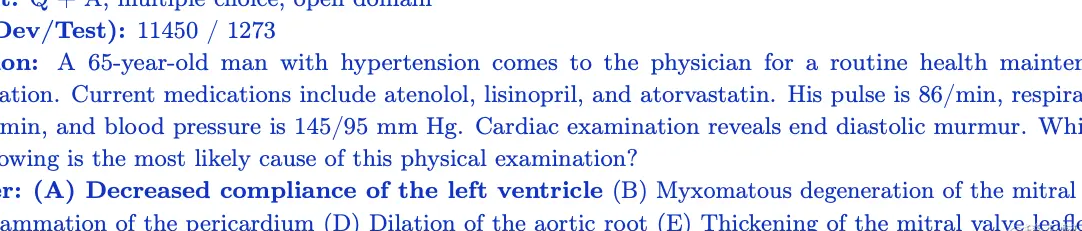
MedMCQA
MedMCQA is a large-scale multiple-choice question and answer dataset derived from the Indian Medical Entrance Test (AIIMS/NEET). It covers 2400 healthcare domain topics and 21 medical subjects with over 187,000 questions in the training set and 6,100 questions in the test set. Each question has 4 answer options with explanations. MedMCQA assesses a model’s general medical knowledge and reasoning abilities.
PubMedQA
PubMedQA is a closed domain question answering dataset where each question can be answered by looking at the relevant context (PubMed abstract). It contains 1,000 expert-labeled question-answer pairs. Each question is accompanied by a PubMed abstract for context, and the task is to provide a yes/no/maybe answer based on the abstract information. The dataset is divided into 500 training questions and 500 test questions. PubMedQA evaluates a model’s ability to understand and reason about scientific biomedical literature.
MMLU subset (Medicine and Biology)
The MMLU benchmark (Measuring Large-Scale Multi-Task Language Understanding) contains multiple-choice questions from various domains. For the open source medical large model rankings, we focus on the subsets most relevant to medical knowledge:
Clinical Knowledge: 265 questions assessing clinical knowledge and decision-making skills.
Medical Genetics: 100 questions covering topics related to medical genetics.
Anatomy: 135 questions assessing knowledge of human anatomy.
Professional Medicine: 272 questions that assess the knowledge required of medical professionals.
College Biology: 144 questions covering college-level biology concepts.
College Medicine: 173 questions assessing college-level medical knowledge.
Each MMLU subset contains multiple-choice questions with 4 answer options designed to assess model understanding of specific medical and biological domains.
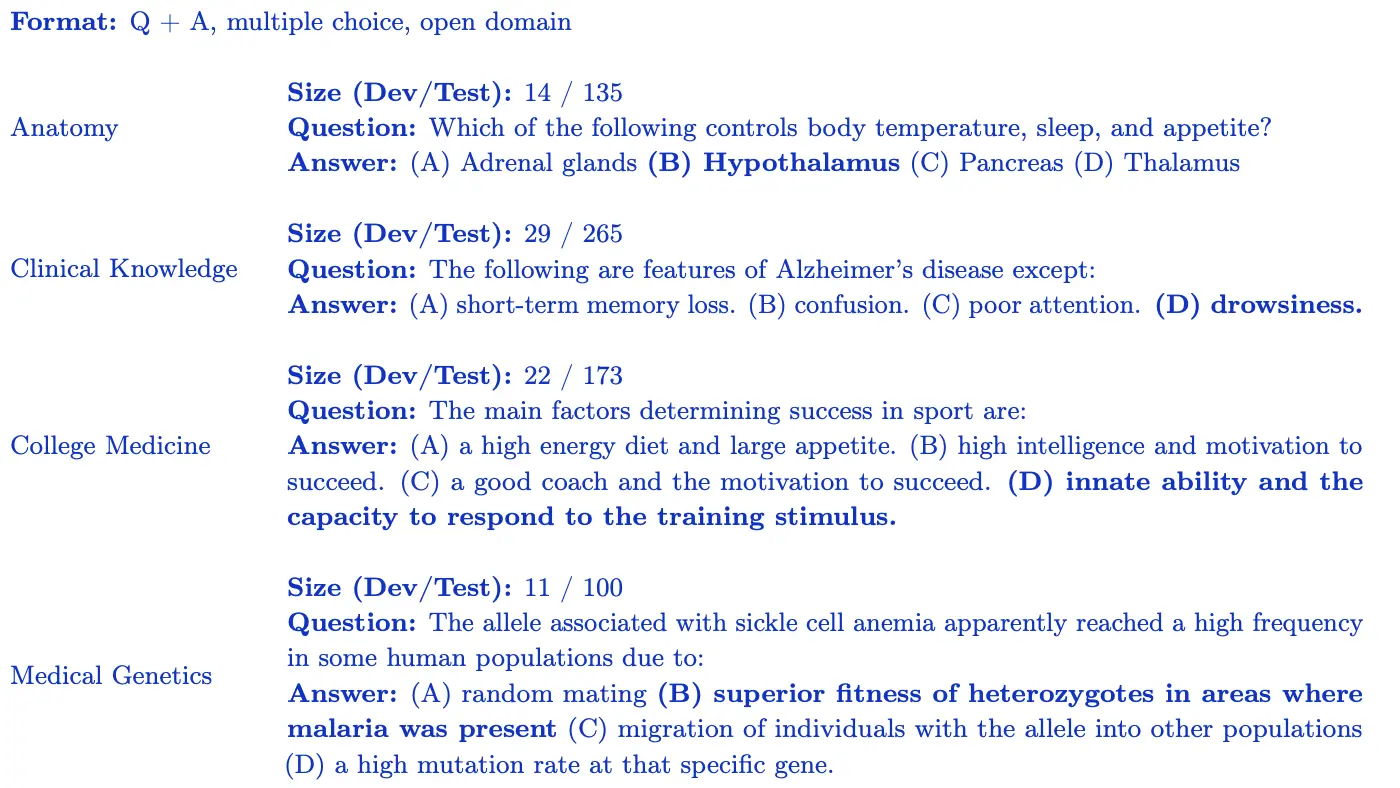
Insights and Analysis
The Open Source Medical Large Model Ranking evaluates the performance of various large language models (LLMs) on a range of medical question answering tasks. Here are some of our key findings:
Commercial models such as GPT-4-base and Med-PaLM-2 consistently achieve high accuracy scores on various medical datasets, demonstrating strong performance in different medical fields.
Open source models, such as Starling-LM-7B, gemma-7b, Mistral-7B-v0.1 and Hermes-2-Pro-Mistral-7B, although the number of parameters is only about 7 billion, perform well on some data sets and tasks. delivered competitive performance.
Commercial and open source models perform well on tasks such as understanding and reasoning about scientific biomedical literature (PubMedQA) and applying clinical knowledge and decision-making skills (MMLU clinical knowledge subset).
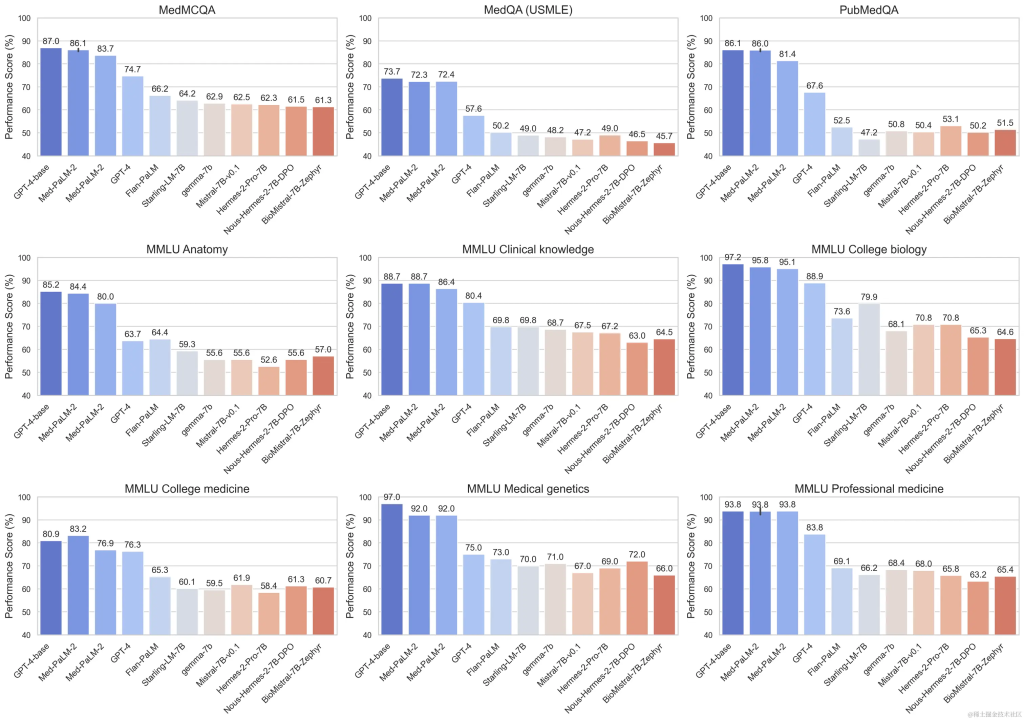
Google’s model, Gemini Pro, has demonstrated strong performance across multiple medical domains, particularly in data-intensive and procedural tasks such as biostatistics, cell biology, and obstetrics and gynecology. However, it showed moderate to low performance in key areas such as anatomy, cardiology, and dermatology, revealing gaps that require further improvements for application in more comprehensive medicine.
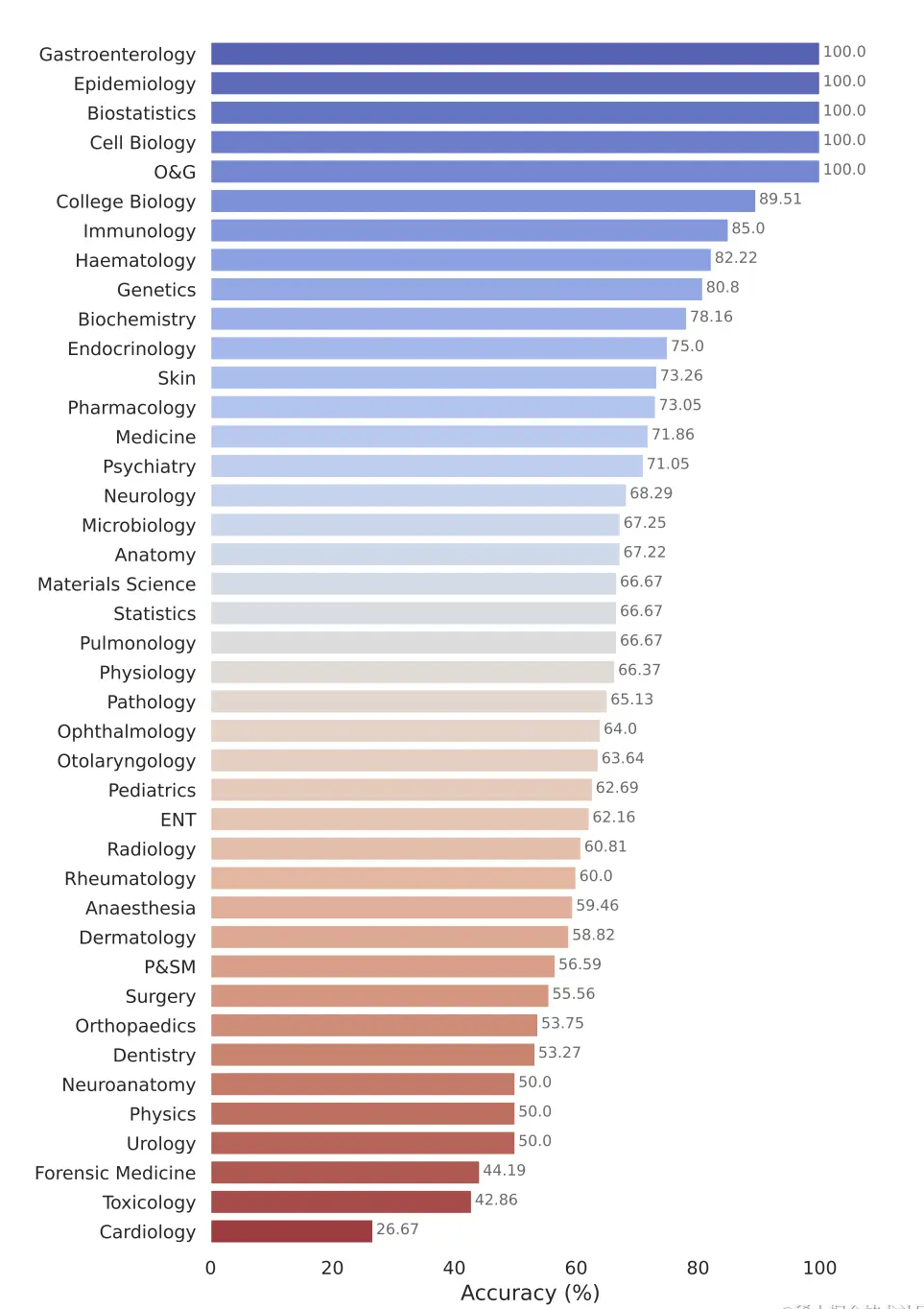
About Open Life Sciences AI
Open Life Sciences AI is a project that aims to revolutionize the use of artificial intelligence in life sciences and healthcare. It serves as a central hub that lists medical models, datasets, benchmarks, and tracks conference deadlines, fostering collaboration, innovation, and advancement in AI-assisted healthcare. We strive to establish Open Life Sciences AI as the premier destination for anyone interested in the intersection of AI and healthcare. We provide a platform for researchers, clinicians, policymakers and industry experts to engage in dialogue, share insights and explore the latest developments in the field.